Our mission
The mission of Qian Research Lab at ShanghaiTech University is to take advantages of engineering informatics to design and develop new multimodal intelligent medical ultrasound, for advancing precise disease diagnosis and treatment. We are a highly interdisciplinary research group with expertise in computer science, electrical system, and medicine. A major focus of our current research is the study and development of advancing imaging technique and artificial intelligence system. Please refer to our publications for an overview of our research.
News
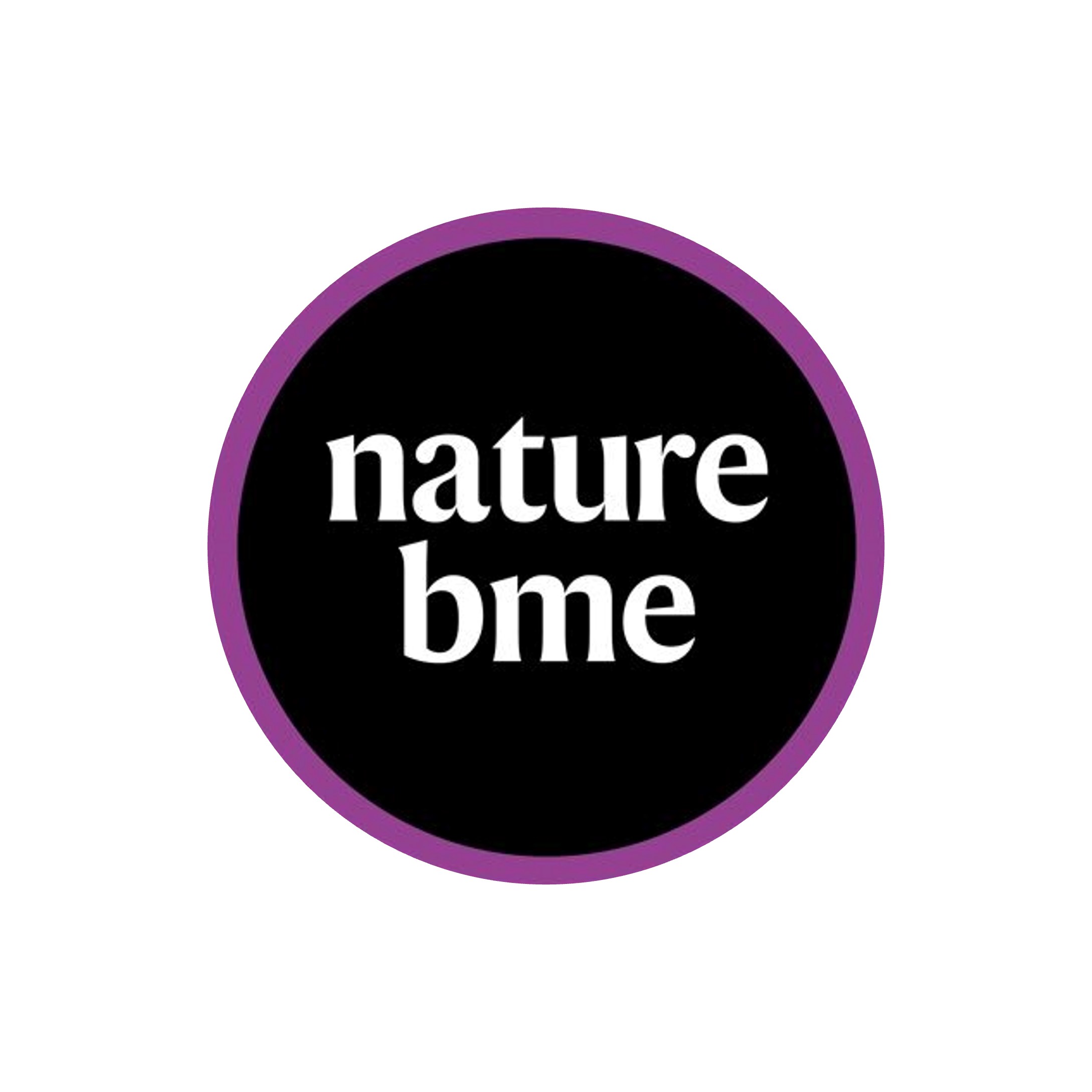
2024.12: New Nature Biomedical Engineering research briefing on “Advancing breast cancer risk stratification using multimodal AI.”
Our work was recognized for its broader significance. For this invited contribution, we introduced our innovative breast disease tree with varying severity levels and cancer risk. Our model was built on the basis of cost-effectiveness and accessibility for cancer screening and diagnosis in real-world clinical settings. It is important to note that breast MRI was excluded as it is mainly used for post-biopsy staging or prognosis.
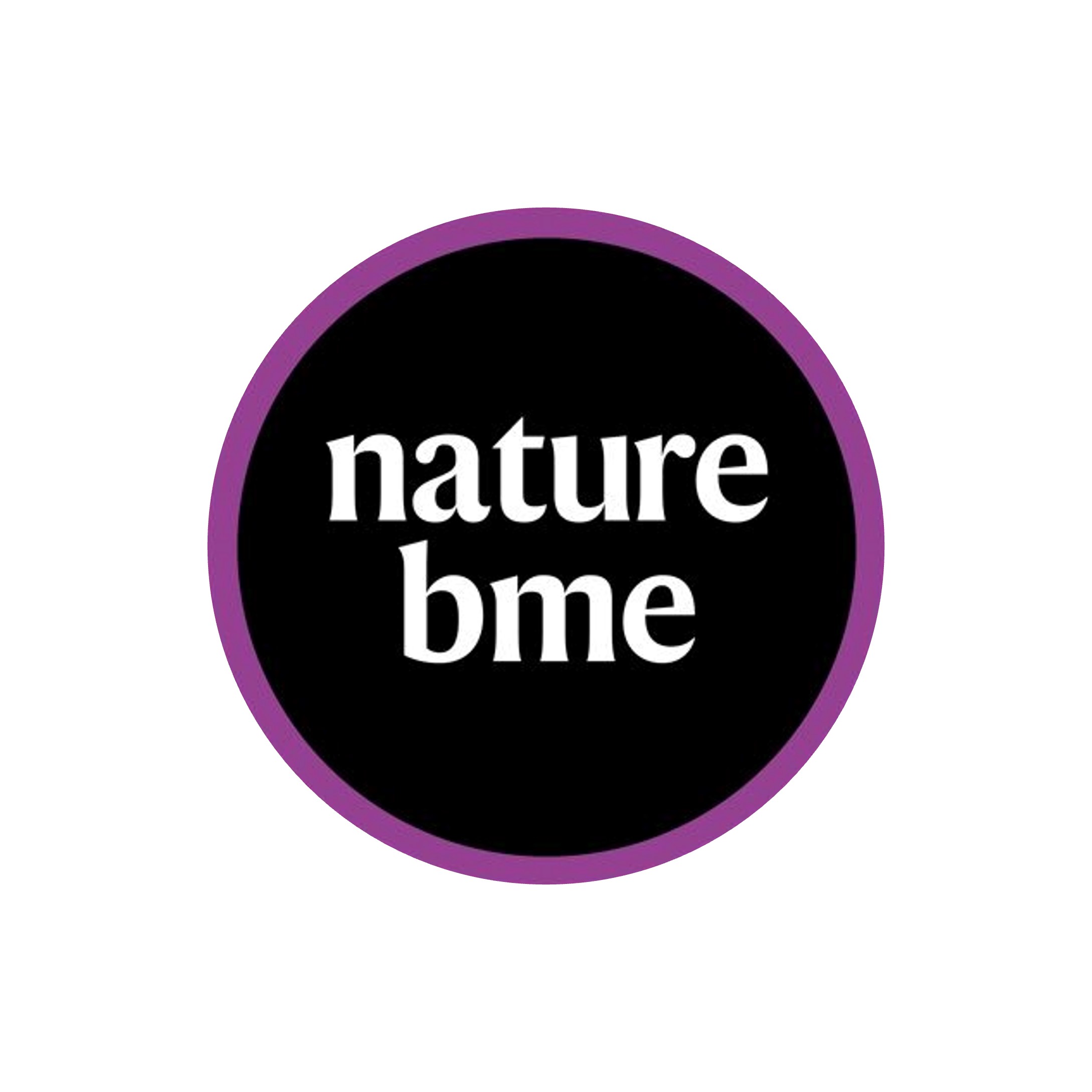
2024.11: New Nature Biomedical Engineering article on “A multimodal machine learning model for the stratification of breast cancer risk.”
In this article, we report a multimodal model for the stratification of breast cancer risk on the basis of clinical metadata, mammography and trimodal ultrasound images. Our BMU-Net model was compared with sonographers, radiologists, pathologists at tumor classification and at differential diagnosis. The results suggest the potential for sub-histopathological differentiation, leading to improved subsequent management strategies.
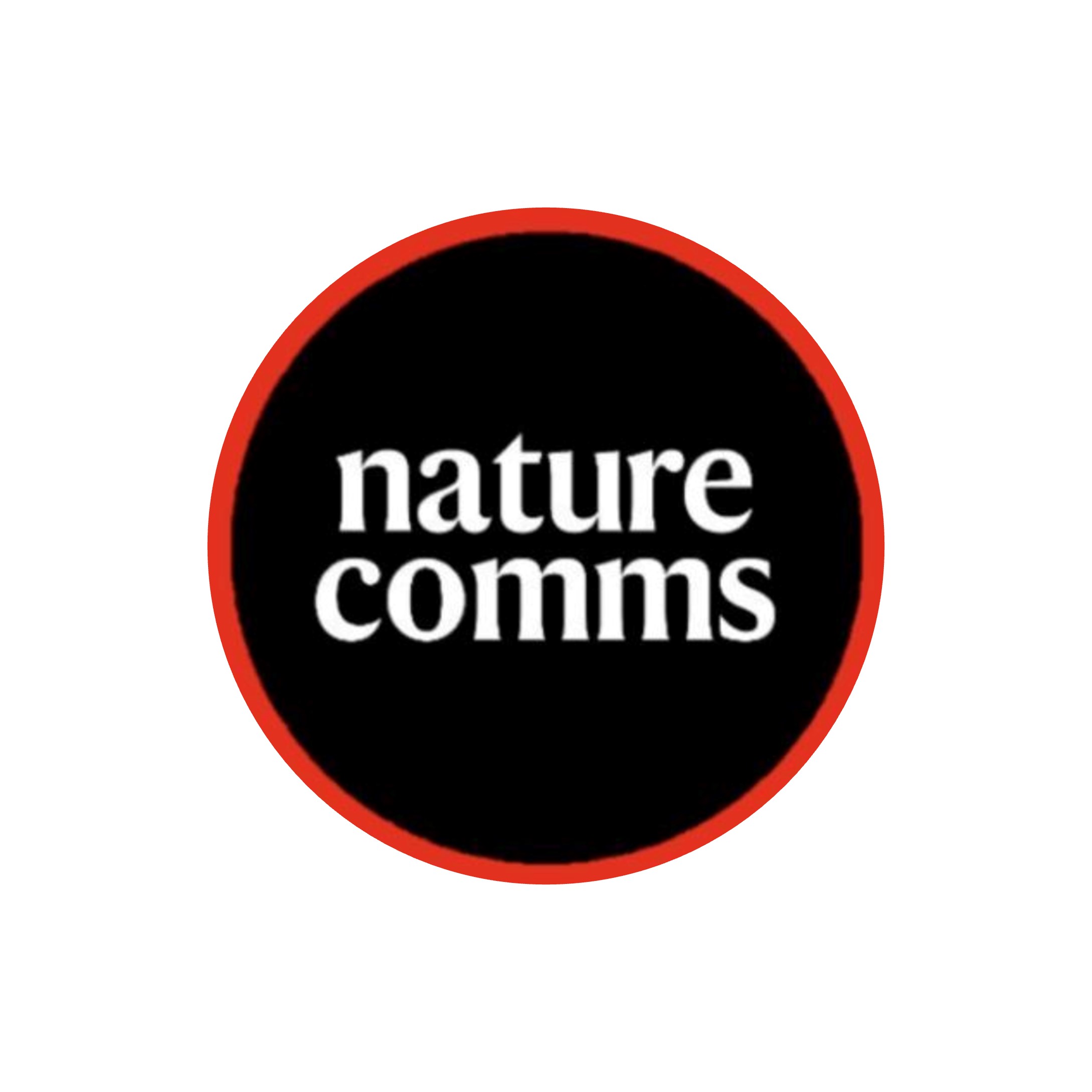
2024.5: New Nature Communications article on “Noninvasive imaging-guided ultrasonic neurostimulation with arbitrary 2D patterns and its application for high-quality vision restoration.”
In this article, we introduce a completely noninvasive ultrasonic retina prosthesis, featuring a customized ultrasound 2D array which allows for simultaneous imaging and stimulation. This is a significant extension of our previous BME Frontiers work on first in vivo blind animal demonstration.
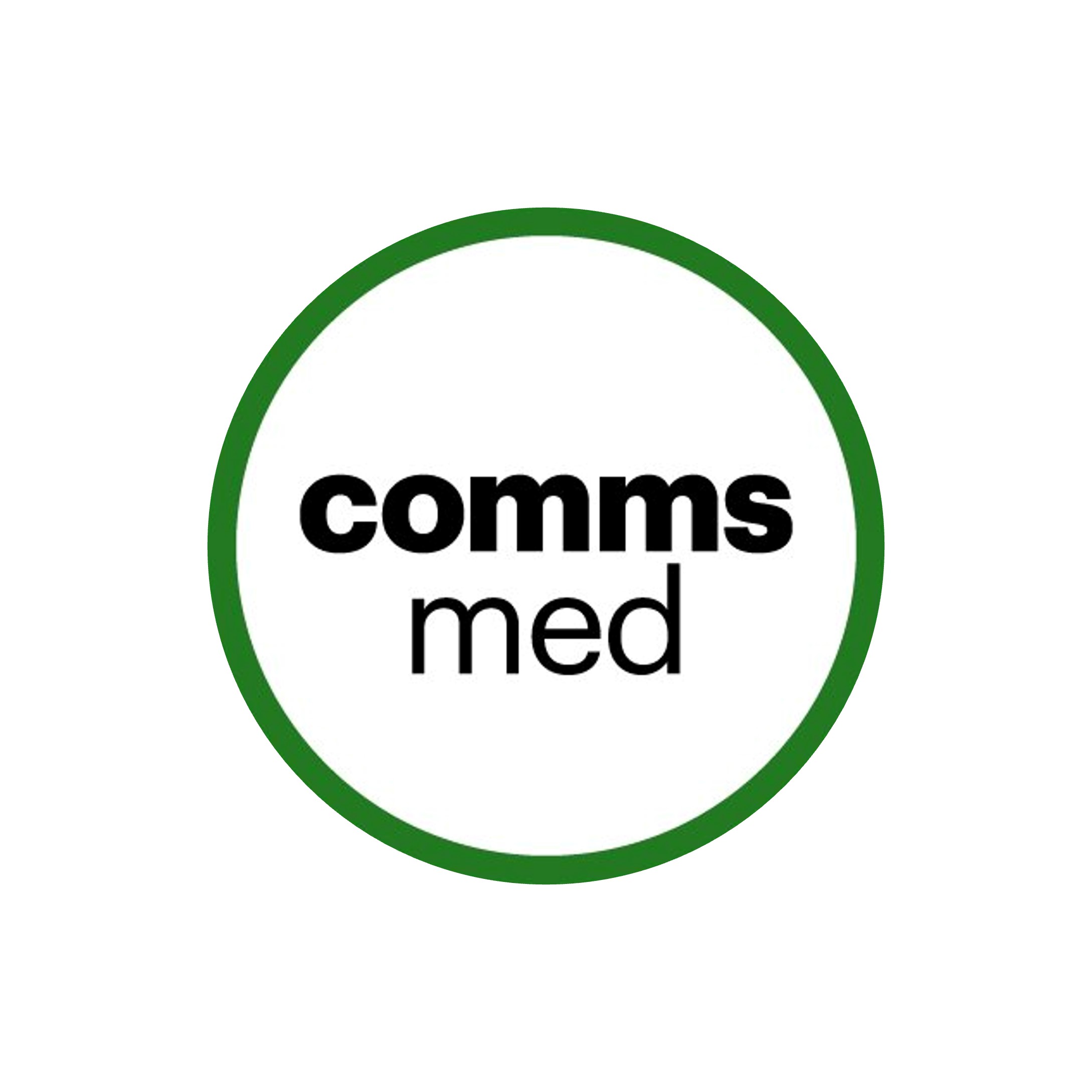
2024.5: New Communications Medicine article on “A domain knowledge-based interpretable deep learning system for improving clinical breast ultrasound diagnosis.”
In this article, we present a MUP-Net model to overcome the black-box nature of deep learning, via enabling doctors to see the reasoning behind the AI’s predictions by visualizing the key image features it analyzed.
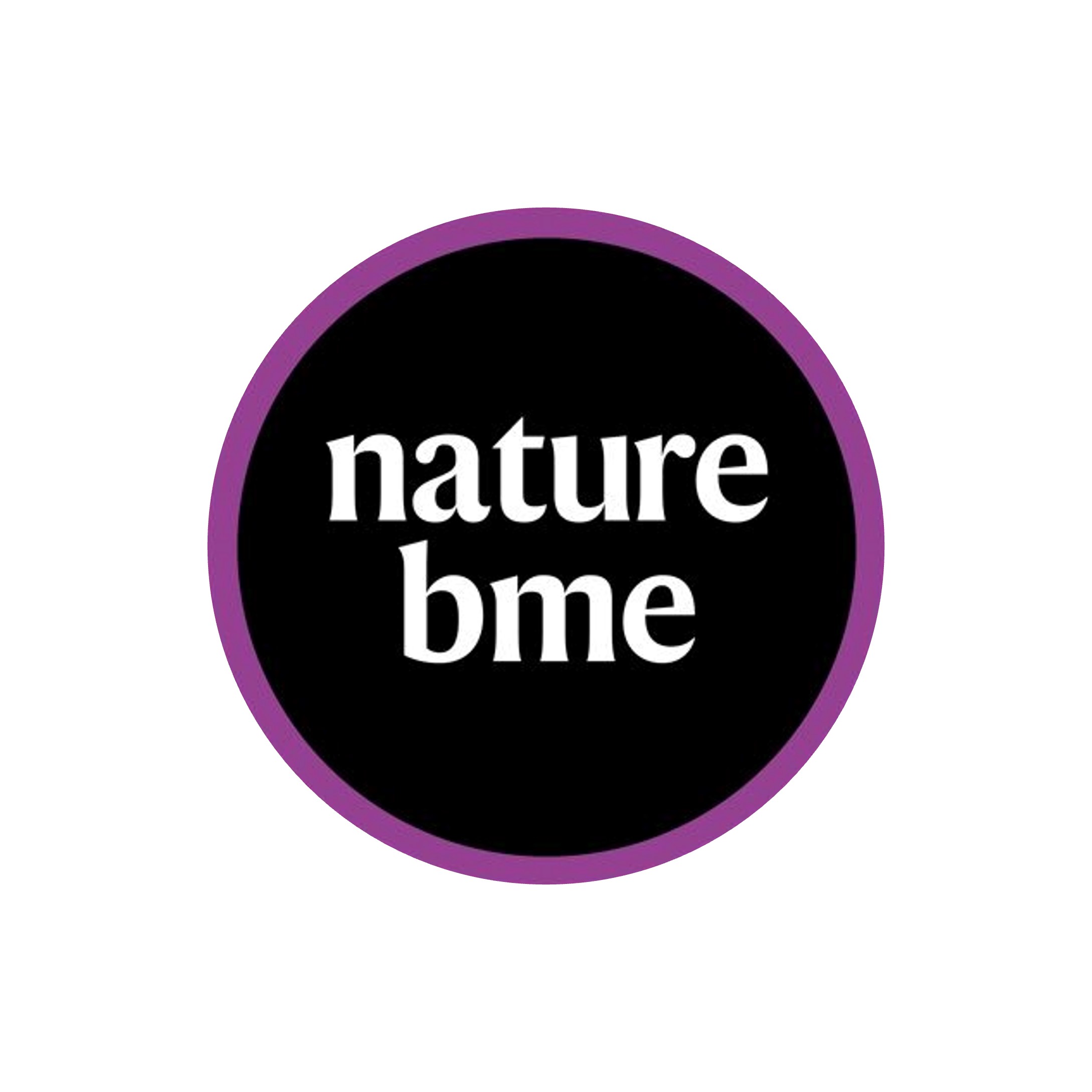
2021.4: New Nature Biomedical Engineering article on “Prospective assessment of breast cancer risk from multimodal multiview ultrasound images via clinically applicable deep learning.”
In this article, we report the first explainable deep learning system to improve breast cancer risk prediction by leveraging multimodal multiview ultrasound images. We compare its performance with radiologists who used BI-RADS in clinical practice and see how their performance improved with AI assistance.